How AI Can Help Reduce Organized Retail Theft
by Moazzam Iqbal on Nov 28, 2024 4:56:22 AM
Learn how AI can help retail stores combat organized theft. Discover cutting-edge AI solutions that improve security, reduce losses, and enhance operational efficiency.
It’s a regular day at your store, but an organized retail theft (ORT) group is already at work. They aren’t just grabbing a few items—they’re strategizing, communicating, and slipping out with thousands of dollars worth of merchandise. You’re facing immediate financial losses and long-term impacts on inventory, sales, and team morale. Traditional security measures – cameras, guards, tags – can’t keep up with these coordinated, sophisticated theft rings. This is where AI for Organized Retail Theft comes in, offering a game-changing, proactive approach to retail theft prevention.
With advanced Retail Theft Prevention Technology, you can identify and prevent ORT incidents before they occur, improving security, reducing losses, and enhancing team efficiency.
The reality is that ORT is on the rise and affecting every aspect of retail operations, from loss prevention to customer satisfaction. But what if you could identify ORT incidents before they happen? What if your security systems could learn and adapt, making prevention more effective over time?
This is where AI comes in—offering a powerful, proactive approach to dealing with organized retail theft.
The Growing Problem of Organized Retail Theft
Organized Retail Theft (ORT) is one of the biggest challenges the retail industry faces today. Unlike opportunistic shoplifting, ORT is a carefully coordinated effort by professional groups.
These groups use tactics that often outpace traditional loss prevention methods, making implementing Retail Theft Prevention Technology essential to stay ahead.
The problem has grown so severe that the sophistication of ORT groups often outpaces traditional loss prevention methods, and retailers are left scrambling for effective solutions to protect their inventory and profits.
What is Organized Retail Theft?
Organized Retail Theft (ORT) involves professional groups planning, coordinating, and executing large-scale thefts across multiple stores and regions. These sophisticated syndicates exploit vulnerabilities in traditional security measures. However, AI for Organized Retail Theft can detect patterns, predict theft, and adapt to evolving tactics, providing a vital tool in combating this growing issue.
For example, ORT groups may assign roles within their teams – some members distract employees while others handle merchandise and transportation. They might target high-value items that can be quickly sold in secondary markets. This level of coordination makes ORT far more challenging to prevent than isolated thefts, as these groups leverage tactics designed to outsmart standard security measures.
The Financial and Operational Impact of Organized Retail Theft
In the U.S. alone, ORT accounts for over $45 billion in losses annually. However, the financial impact only scratches the surface. ORT affects employee morale, disrupts inventory management, and critically impacts customer experience.
Retail managers are forced to increase prices and absorb high-security costs when items are frequently stolen. This “security overload” can push away customers and harm brand reputation.
Retailers also face operational disruptions due to ORT. For example, frequent thefts lead to inventory imbalances, complicated order fulfillment and sales forecasting. Employees may feel demoralized, too, as they repeatedly encounter the effects of theft, which can lead to higher turnover and further challenges in maintaining store operations.
Why Traditional Methods Falling Short
Most stores rely on a combination of security guards, surveillance cameras, and tagging systems. But ORT syndicates are usually a step ahead, exploiting the limitations of these methods. Cameras are passive observers – they document but don’t deter. Tags can be removed. Guards, while essential, can’t monitor every inch of a store or recognize patterns across locations. Traditional methods are reactive, not proactive.
As a result, retail security teams often find themselves playing catch-up, responding to thefts only after they’ve occurred. The lack of predictive or preventive capabilities in traditional security solutions means ORT groups have a considerable advantage.
Retailers need a more innovative, more adaptable approach to combat the evolving tactics of ORT groups effectively.
Why the Status Quo Isn’t Working: The Need for Advanced Solutions
With the growing sophistication of ORT groups, the traditional methods retailers rely on are no longer enough. The reactive approach of basic surveillance and security protocols often allows ORT groups to operate without facing real deterrents.
Retailers are finding that they must go beyond simple guards and cameras if they hope to stay a step ahead of these criminals.
Retail loss prevention teams are stretched thin, managing multiple store locations, coordinating responses, and dealing with increasing numbers of incidents. The challenges of trying to monitor, review, and respond manually to each potential incident are overwhelming. Without a solution that proactively addresses ORT, teams are left constantly reacting to thefts instead of preventing them.
The Increasing Sophistication of Organized Retail Theft Groups
ORT groups have evolved. Today, they use encrypted communication, coordinate on social media, and exploit store vulnerabilities with precision. These tactics make ORT a constant and evolving threat. Retailers today are playing catch-up in a game where the stakes keep rising.
For example, some ORT groups communicate through encrypted apps or use coded language on social media to avoid detection. They may conduct reconnaissance missions to identify weaknesses in security and store layouts.
With these increasingly complex tactics, retailers need advanced solutions capable of adapting to and countering the growing sophistication of ORT activities.
The Pressure on Retail Security Teams
Retail loss prevention teams find it exhausting to coordinate security across dozens of stores, each with unique layouts and vulnerabilities. Manually reviewing footage, analyzing patterns, and responding to incidents is a slow, labor-intensive process. Teams are overwhelmed and understaffed, leading to burnout and higher costs.
As ORT incidents increase, the demand for security teams grows, but with limited tools, they struggle to respond effectively. Many stores operate with minimal resources, unable to provide dedicated personnel to continuously monitor security footage or identify trends across multiple locations.
Organized Retail Theft Impacts Retail Profits and Customer Experience
Each stolen product either adds to retailer losses or increases customer prices. ORT impacts customer experience by making stores feel less welcoming, as heightened security can deter honest customers. ORT doesn’t just threaten security – it undermines the entire retail experience.
Frequent theft incidents can create a tense environment, leading to heightened surveillance and store policies that make honest customers feel scrutinized. This can erode customer trust and loyalty, further impacting the retailer’s bottom line.
How AI Can Help Combat Organized Retail Theft
AI is transforming loss prevention, shifting from reactive to proactive. Instead of just observing or recording incidents, AI uses data to predict, prevent, and reduce them. AI-powered solutions help retailers leverage machine learning, predictive analytics, and computer vision to enhance security efficiently.
These technologies allow AI to analyze patterns and identify risks in real-time, enabling loss prevention teams to address issues before they escalate. By automating monitoring and providing predictive insights, AI frees up resources for security teams, allowing them to focus on higher-value tasks.
The Role of AI in Loss Prevention
AI plays a pivotal role in reshaping retail security strategies. Through machine learning and data analytics, AI systems can analyze historical theft data, identify patterns, and predict where and when ORT incidents are likely to occur.
This enables a shift from reactive security responses to preventive measures, making it harder for ORT groups to operate undetected.
For instance, AI can alert security teams about unusual activity patterns, such as groups frequently visiting high-value sections or loitering in specific areas, allowing for preemptive intervention.
Key Areas Where AI Reduces ORT
AI combats organized retail theft (ORT) by enhancing store security through real-time analytics, predictive capabilities, and integrated data across locations. This multi-faceted approach enables retailers to monitor, predict, and prevent theft more effectively than traditional methods, targeting key prevention, detection, and response areas.
Real-Time Video Analytics and Surveillance
AI-powered activity recognition combats organized retail theft by spotting suspicious behaviors in real-time—such as coordinated item sweeps or loitering in high-risk zones—and sending immediate alerts to security. By tracking patterns, AI can identify repeat offenders, enabling proactive monitoring.
It also adapts to new theft tactics, learning from behavior trends to detect emerging methods like distraction schemes. Lastly, AI insights inform smarter store layouts and product placement, reducing vulnerabilities to theft rings.
For example, AI can detect two or three individuals loitering in high-value sections without purchasing, signaling potential ORT behavior. AI’s real-time analysis makes video surveillance an active security tool rather than a passive recorder.
Inventory Management and Predictive Analytics
AI goes beyond monitoring; it excels at predicting patterns based on historical data. By analyzing data such as seasonal trends and store-specific factors, AI can forecast when and where theft will likely occur, allowing security teams to allocate resources proactively.
Predictive analytics also allow stores to adjust staffing and security based on real-time data rather than guesswork.
This ability to predict high-risk periods or locations ensures that security teams are always one step ahead, deploying resources effectively and minimizing ORT incidents.
Data Integration and Inter-store Collaboration
AI enables data integration, creating a unified database of theft patterns across stores or retail chains. This shared intelligence helps identify ORT networks and coordinate responses. If a store experiences a surge in ORT, AI can alert nearby stores, allowing them to take preventive measures.
By centralizing data across multiple locations, AI enables inter-store collaboration, making it easier to track ORT groups that target multiple stores in a region and implement coordinated responses.
Facial Recognition and Behavioral Analytics
Facial recognition technology helps AI identify known ORT members based on shared databases or customer histories. Combined with behavioral analytics, AI can alert staff if a potential repeat offender is detected. Privacy is a valid concern, but many AI tools offer anonymization and transparency, allowing ethical and effective ORT prevention.
Integrating facial recognition with behavioral analytics gives retailers a proactive way to identify potential ORT members without compromising customer privacy, as many systems allow for ethical data handling.
Real-World Examples of AI in Action
Case studies highlight AI’s effectiveness. For instance, a national retailer that deployed AI-driven video analytics saw a 30% reduction in ORT incidents within the first year. Another retailer implemented facial recognition in high-risk locations, identifying and preventing repeat offenders and saving hundreds of thousands annually.
These examples illustrate AI solutions' concrete, measurable impact on reducing ORT, proving that AI-driven tools are valuable investments in retail security.
Addressing Concerns Around AI Implementation
Implementing AI-driven security can raise concerns around privacy, cost, and team acceptance. Addressing these concerns is critical to ensure a smooth adoption of AI in retail security.
Compliance and Ethical Considerations
AI-powered redaction tools are becoming essential for retailers tackling organized retail theft (ORT) and enhancing security while meeting privacy and compliance standards like HIPAA, FOIA, and CJIS.
These tools automatically redact Personally Identifiable Information (PII)—including faces and license plates—allowing retailers to securely share footage with law enforcement without compromising customer privacy.
HIPAA compliance safeguards sensitive health data, FOIA ensures the sharing of secure public records, and CJIS protects criminal justice information. This approach minimizes legal risks and maintains secure management of footage containing sensitive information related to ORT incidents.
Privacy concerns, especially with facial recognition in theft prevention, underscore the need for ethical AI practices. Responsible AI use involves anonymizing data, providing opt-in options, and maintaining transparent data handling.
Retailers should collaborate with AI providers to set guidelines prioritizing customer security and respect, ensuring customers feel protected while addressing ORT. Choosing ethically driven solutions enables retailers to combat ORT effectively while upholding customer trust and respecting privacy rights.
Cost and Resource Investment
Initial setup costs for AI solutions can be high, but the long-term ROI is substantial. For hesitant stores, phased implementation and cloud-based services make AI more affordable. AI-as-a-service models allow retailers to pay for what they need, making AI accessible and scalable.
These cost models ensure that even smaller retailers can benefit from AI-driven security without overwhelming budgets.
Overcoming Staff Resistance
AI adoption can trigger resistance among employees who are concerned about job security. Retailers can alleviate these concerns by emphasizing that AI enhances, not replaces, security teams. AI automates data-heavy tasks, allowing staff to focus on higher-level analysis and improving efficiency and security.
With proper training and communication, retailers can show their teams that AI is a tool to help them, not replace them.
The Future of AI in Retail Security
The role of AI in retail security is set to grow, offering exciting innovations and opportunities for further collaboration across the industry.
AI Innovations on the Horizon
Emerging AI technologies, like autonomous drones and advanced predictive models, are shaping the future of retail security. These innovations will allow retailers to deploy mobile, autonomous monitoring solutions, enhancing coverage without overwhelming staff.
These tools will continue to strengthen AI’s ability to monitor, predict, and prevent ORT in more sophisticated ways.
Expansion of AI Solutions Across Retail Channels
AI’s role is expanding beyond physical stores to online and hybrid retail channels. AI can help retailers protect physical and digital assets, making it a valuable tool in the fight against organized crime across channels.
With omnichannel integration, AI-driven security solutions create a seamless protection framework for retailers operating across multiple channels.
Industry-Wide Collaboration Potential
AI’s future in retail security includes the potential for industry-wide collaboration. Shared databases and information networks could help retailers, law enforcement, and other stakeholders work together to prevent ORT. By sharing data, retailers can tackle ORT more effectively.
Collaborative AI databases could help retailers track and prevent organized crime on a larger scale, benefiting the industry.
Conclusion
AI is proving to be a game-changing tool in retail loss prevention. By transforming security from reactive to proactive, AI helps retailers reduce organized retail theft, save on costs, and improve the shopping experience. As ORT tactics evolve, AI’s adaptability ensures that retailers stay one step ahead.
Adopting AI for Organized Retail Theft prevention is an investment in security, customer satisfaction, operational efficiency, and long-term profitability.
People also ask
How does AI detect organized retail theft (ORT) in real-time?
AI uses real-time video analytics and machine learning algorithms to detect unusual patterns, such as group formations, frequent visits to high-value sections, or erratic behavior. This allows security teams to intervene proactively, often preventing theft.
Is implementing AI for retail theft prevention costly?
Initial setup costs may be high, but the long-term ROI is substantial due to reductions in theft and improvements in efficiency. Many AI solutions offer scalable, cloud-based models, making adoption accessible and cost-effective.
How does AI help with inventory management to prevent Organized Retail Theft?
AI-based predictive analytics forecast theft patterns based on historical data, helping teams proactively secure high-risk products and areas. This reduces ORT incidents and enhances inventory accuracy.
Can AI facial recognition identify repeat offenders, and is it ethical?
Yes, AI can identify repeat offenders, but ethical use is crucial. Many solutions anonymize data and provide transparency, allowing adequate security while respecting customer privacy.
How does AI integrate with existing security systems?
AI solutions integrate with existing camera systems, POS data, and inventory management systems, creating a unified theft detection and prevention platform. This transforms passive systems into proactive security assets.
Will AI replace human security teams in retail?
AI is a tool to enhance, not replace, human teams. AI automates data-heavy tasks, allowing staff to focus on high-level analysis and customer service, making security teams more effective.
Can AI share data across multiple stores for better Organized Retail Theft prevention?
Yes, AI centralizes data across locations, creating a shared intelligence network that helps identify ORT patterns on a broader scale. This enables multiple stores to detect trends and take proactive measures.
Jump to
You May Also Like
These Related Stories
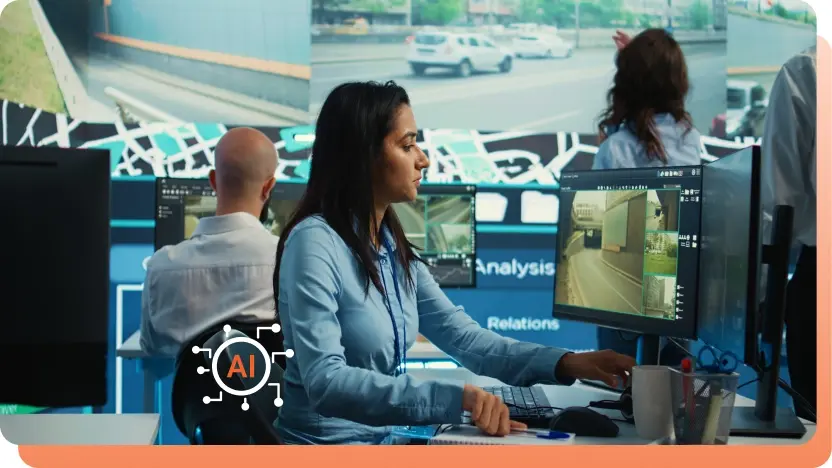
Intelligence-Led Policing in the Era of Artificial Intelligence
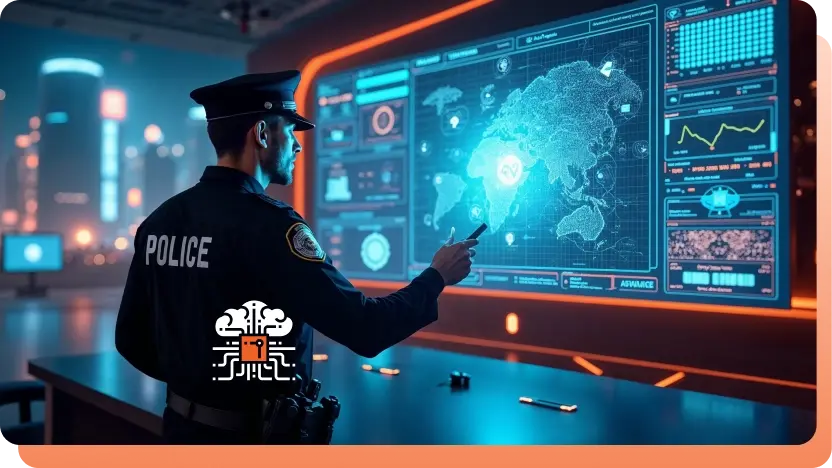
Future Trends in AI-Driven Law Enforcement
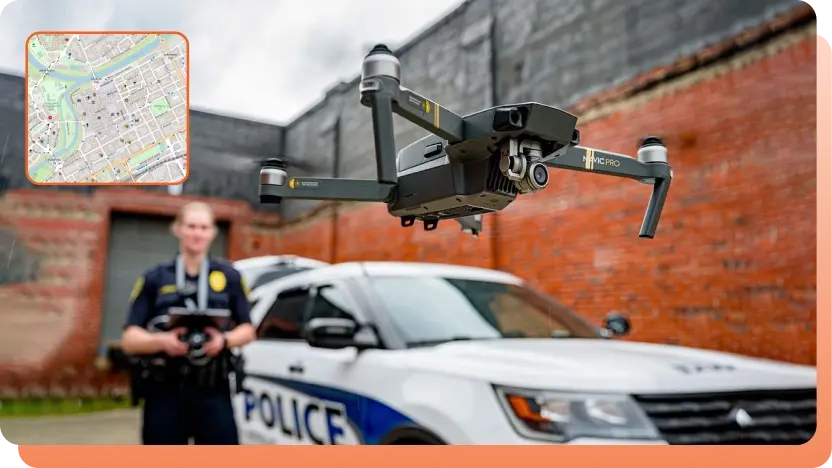
No Comments Yet
Let us know what you think